About The Workshop
Novel applications of affective computing have emerged in recent years in domains ranging from health care to the 5th generation mobile network. Many of these have found improved emotion classification performance when fusing multiple sources of data (e.g., audio, video, brain, face, thermal, physiological, environmental, positional, text, etc.). Multimodal affect recognition has the potential to revolutionize the way various industries and sectors utilize information gained from recognition of a person's emotional state, particularly considering the flexibility in the choice of modalities and measurement tools (e.g., surveillance versus mobile device cameras). Multimodal classification methods have been proven highly effective at minimizing misclassification error in practice and in dynamic conditions. Further, multimodal classification models tend to be more stable over time compared to relying on a single modality, increasing their reliability in sensitive applications such as mental health monitoring and automobile driver state recognition. To continue the trend of lab to practice within the field and encourage new applications of affective computing, this workshop provides a forum for researchers to exchange ideas on future directions, including novel fusion methods and databases, innovations through interdisciplinary research, and emerging emotion sensing devices. Also, this workshop places a focus on the ethical use of novel applications of affective computing in real world scenarios. More specifically, it welcomes discussions on topics including, but not limited to, privacy, manipulation of users, and public fears and misconceptions regarding affective computing. It is expected that the affective computing market will grow from $28.6 billion to $140 billion by 2025. This significant growth will allow for new applications into affective computing that include, but are not limited to, health monitoring systems, diagnosis and treatment of disorders such as Autism Spectrum Disorder, and home entertainment (e.g., video games). To improve these affective systems, there are many ethical concerns to be considered. This workshop seeks to explore the intersection between theory and ethical applications of affective computing, with a specific focus on multimodal data for affect recognition (e.g., expression, and physiological signals).
Keynote Speakers
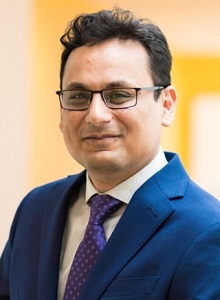
Ehsan Hoque
Dr. Ehsan Hoque is an associate professor of computer science at the University of Rochester, where he co-leads the Rochester Human-Computer Interaction (ROC HCI) Group. From 2018-2019, he was also the Interim Director of the Goergen Institute for Data Science. Ehsan earned his Ph.D. from MIT Media Lab in 2013, where the MIT Museum highlighted his dissertation—the development of an intelligent agent to improve human ability — as one of MIT's most unconventional inventions. Building on the work/patent, Microsoft released "Presenter Coach" in 2019 to be integrated into PowerPoint. Ehsan is best known for introducing and extensively validating the idea of using AI to train and enhance elements of basic human ability.
Dr. Ehsan Hoque is an associate professor of computer science at the University of Rochester, where he co-leads the Rochester Human-Computer Interaction (ROC HCI) Group. From 2018-2019, he was also the Interim Director of the Goergen Institute for Data Science. Ehsan earned his Ph.D. from MIT Media Lab in 2013, where the MIT Museum highlighted his dissertation—the development of an intelligent agent to improve human ability — as one of MIT's most unconventional inventions. Building on the work/patent, Microsoft released "Presenter Coach" in 2019 to be integrated into PowerPoint. Ehsan is best known for introducing and extensively validating the idea of using AI to train and enhance elements of basic human ability.
Ehsan and his students' work has been recognized by NSF CAREER Award, MIT TR35, Young Investigator Award by the US Army Research Office (ARO). In 2017, Science News named him one of the 10 scientists to watch, and in 2020, the National Academy of Medicine recognized him as one of the emerging leaders in health and medicine. Ehsan is an inaugural member of the ACM's Future of Computing Academy.
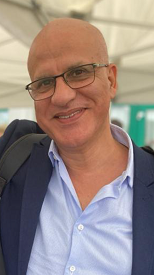
Mohamed Daoudi
Dr. Mohamed Daoudi is a Full Professor of Computer Science at IMT Lille Douai and the Head of Image group at CRIStAL Laboratory (UMR CNRS 9189). He received his Ph.D. degree in Computer Engineering from the University of Lille (France) in 1993. My main interest lies in the use of computer and pattern recognition in human behavior understanding. He has published over 150 papers in some of the most distinguished scientific journals and international conferences. He is Associate Editor of Image and Vision Computing Journal, IEEE Transactions on Multimedia, Computer Vision and Image Understanding and Journal of Imaging. He was the General Chair of IEEE International Conference on Automatic Face and Gesture Recognition, 2019. He is Fellow of IAPR and IEEE Senior member.
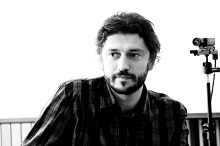
Michel Valstar
Dr. Michel Valstar is a Professor of Computer Science and the University of Nottingham. He is a researcher in Automatic Visual Understanding of Human Behaviour. This encompasses Machine Learning, Computer Vision, and a good idea of how people behave in this world.
More details coming soon.
Workshop Schedule
2:00P-2:10P BST | Welcome and Opening Remarks |
2:15P-3:00P BST | Keynote 1 - Michel Valstar |
3:00P-3:15P BST | S. Samrose and E. Hoque – Quantifying the Intensity of Toxicity for Discussion and Speakers |
3:15P-3:30P BST | I. Tynes and S. Canavan – Real-time Ubiquitous Pain Recognition |
3:30P-3:40P BST | Coffee Break 1 |
3:40P-4:25P BST | Keynote 2 - Ehsan Hoque Multimodal Analysis to Ensure Equity and Access in Healthcare By 2030, the old will begin to outnumber the young for the first time in recorded history. Population aging is poised to impose a significant strain on economies, health systems, and social structures. However, it also presents a unique opportunity for affective computing to introduce personalization and inclusiveness to ensure equity in aging. Vulnerable populations such as older adults learn, trust, and use new technologies differently. Any prediction algorithm we develop must use high-quality and population-representative input data outside of the clinic and produce accurate, generalizable, and unbiased results. Therefore, the translational path for multimodal affect analysis into clinical care needs deeper engagement with all the stakeholders to solve a pressing problem with a practical solution that end-users, clinicians, and patients all find value in. In this talk, I will provide some examples of working systems evaluated by controlled experiments and could potentially be deployed in the real world to ensure equity and access among the aging population. In particular, I will highlight two specific examples: 1) Innovating for Parkinson's, the fastest-growing neurological disability and is linked with aging. 2) Modeling end-of-life communication with terminal cancer patients where their values and preferences are respected as they plan for a deeply personal human experience such as death. |
4:25P-4:40P BST | W. Rahman, S. Mahbub, A. Salekin, Md K. Hasan and E. Hoque - HirePreter: A Framework for Providing Fine-grained Interpretation for Automated Job Interview Analysis |
4:40P-4:55P BST | A. Bhatti, B. Behinaein, D. Rodenburg, P. Hungler and A. Etemad - Attentive Cross-modal Connections for Deep Multimodal Wearable-based Emotion Recognition |
4:55P-5:05P BST | Coffee Break 2 |
5:05P-5:50P BST | Keynote 3 - Mohamed Daoudi Face and Body Shape Analysis with Application in Emotion Recognition and Well-Being Face and body shape analysis is attracting great interest for potential applications in a multitude of real-life situations. Much of the Computer Vision research in this field has focused on relating facial expressions analysis, with investigations rarely including more than upper body. In this talk, I will present my research team’s explorations specifically in the area of face and body shape with applications in emotion recognition and well-being. |
5:50P-6:00P BST | Defining Breakout Groups |
6:00P-6:45P BST | Breakout Group Meetings |
6:45P-7:30P BST | Breakout Group Reporting |
7:30P-End BST | Closing/Compilation of Breakout Group Topics for Submission to AAAC |
Call for Papers
To investigate ethical, applied affect recognition, this workshop will leverage multimodal data that includes, but is not limited to, 2D, 3D, thermal, brain, physiological, and mobile sensor signals. This workshop aims to expose current use cases for affective computing and emerging applications of affective computing to spark future work. Along with this, this workshop has a specific focus on the ethical considerations of such work, including how to mitigate ethical concerns. Considering this, topics of the workshop will focus on questions including, but not limited to:
- What inter-correlations exist between facial affect (e.g. expression) and other modalities (e.g. EEG)?
- How can multimodal data be leveraged to create real-world applications of affect recognition such as prediction of stress, real-time ubiquitous emotion recognition, and impact of mood on ubiquitous subject identification?
- How can we facilitate the collection of multimodal data for applied affect recognition?
- What are the ethical implications of working on such questions?
- How can we mitigate the ethical concerns that such work produces?
- Can we positively address public fears and misconceptions regarding applied affective computing?
- Health applications with a focus on multimodal affect
- Multimodal affective computing for cybersecurity applications (e.g., biometrics and IoT security)
- Inter-correlations and fusion of ubiquitous multimodal data as it relates to applied emotion recognition (e.g. face and EEG data)
- Leveraging ubiquitous devices to create reliable multimodal applications for emotion recognition
- Applications of in-the-wild data vs. lab controlled
- Facilitation and collection of multimodal data (e.g. ubiquitous data) for applied emotion recognition
- Engineering applications of multimodal affect (e.g., robotics, social engineering, domain inspired hardware / sensing technologies, etc.)
- Privacy and security
- Institutionalized bias
- Trustworthy applications of affective computing
- Equal access to ethical applications of affective computing (e.g. medical applications inaccessible due to wealth inequality)
Workshop candidates are invited to submit papers up to 4 pages plus one for references in the ACII format. Submissions to AMAR 2021 should have no substantial overlap with any other paper submitted to ACII2021 or already published. All persons who have made any substantial contribution to the work should be listed as authors (in the accepted version), and all listed authors should have made some substantial contribution to the work. Papers presented at AMAR 2021 will appear in the IEEE Xplore digital library. Papers should follow the ACII conference format (anonymous).
How to Submit:
Paper submissions will be handled using EasyChair. Select the "ACII 2021 Workshop - Applied Multimodal Affect Recognition" track. The reviewing process will be double blind. Authors should remove author and institutional identities from the title and header areas of the paper. There should also be no acknowledgments. Authors can leave citations to their previous work unanonymized so that reviewers can ensure that all previous research has been taken into account. However, they should cite their own work in the third person (e.g., "[25] found that…"). At least one author of each accepted paper will be required to attend the workshop to present their work.
Important dates:
Paper submission: June 30, 2021
Decision to Authors: July 14, 2021
Camera-ready papers due: July 28, 2021
Workshop: September 28, 2021
Accepted Papers
Real-time Ubiquitous Pain Recognition
Iyonna Tynes and Shaun Canavan
Emotion recognition is a quickly growing field due to the increased interest in building systems which can classify and respond to emotions. Recent medical crises, such as the opioid overdose epidemic in the United States and the global COVID-19 pandemic has emphasized the importance of emotion recognition applications is areas like Telehealth services. Considering this, we propose an approach to real-time ubiquitous pain recognition from facial images. We have conducted offline experiments using the BP4D dataset, where we investigate the impact of gender and data imbalance. This paper proposes an affordable and easily accessible system which can perform pain recognition inferences. The results from this study found a balanced dataset, in terms of class and gender, results in the highest accuracies for pain recognition. We also detail the difficulties of pain recognition using facial images and propose some future work that can be investigated for this challenging problem.
Attentive Cross-modal Connections for Deep Multimodal Wearable-based Emotion Recognition
Anubhav Bhatti, Behnam Behinaein, Dirk Rodenburg, Paul Hungler and Ali Etemad
Classification of human emotions can play an essential role in the design and improvement of human-machine systems. While individual biological signals such as Electrocardiogram (ECG) and Electrodermal Activity (EDA) have been widely used for emotion recognition with machine learning methods, multimodal approaches generally fuse extracted features or final classification/regression results to boost performance. To enhance multimodal learning, we present a novel attentive cross-modal connection to share information between convolutional neural networks responsible for learning individual modalities. Specifically, these connections improve emotion classification by sharing intermediate representations among EDA and ECG and apply attention weights to the shared information, thus learning more effective multimodal embeddings. We perform experiments on the WESAD dataset to identify the best configuration of the proposed method for emotion classification. Our experiments show that the proposed approach is capable of learning strong multimodal representations and outperforms a number of baselines methods.
HirePreter: A Framework for Providing Fine-grained Interpretation for Automated Job Interview Analysis
Wasifur Rahman, Sazan Mahbub, Asif Salekin, Md Kamrul Hasan and Ehsan Hoque
There has been a rise in automated technologies to screen potential job applicants through affective signals captured from video-based interviews. These tools can make the interview process scalable and objective, but they often provide little to no information of how the machine learning model is making crucial decisions that impacts the livelihood of thousands of people. We built an ensemble model -- by combining Multiple-Instance-Learning and Language-Modeling based models -- that can predict whether an interviewee should be hired or not. Using both model-specific and model-agnostic interpretation techniques, we can decipher the most informative time-segments and features driving the model’s decision making. Our analysis also shows that our models are significantly impacted by the beginning and ending portions of the video. Our model achieves 75.3% accuracy in predicting whether an interviewee should be hired on the ETS Job Interview dataset. Our approach can be extended to interpret other video-based affective computing tasks like analyzing sentiment, measuring credibility, or coaching individuals to collaborate more effectively in a team.
Quantifying the Intensity of Toxicity for Discussions and Speakers
Samiha Samrose and Ehsan Hoque
In this work, from YouTube News-show multimodal dataset with dyadic speakers having heated discussions, we analyze the toxicity through audio-visual signals. Firstly, as different speakers may contribute differently towards the toxicity, we propose a speaker-wise toxicity score revealing individual proportionate contribution. As discussions with disagreements may reflect some signals of toxicity, in order to identify discussions needing more attention we categorize discussions into binary high-low toxicity levels. By analyzing visual features, we show that the levels correlate with facial expressions as Upper Lid Raiser (associated with 'surprise'), Dimpler (associated with 'contempt'), and Lip Corner Depressor (associated with 'disgust') remain statistically significant in separating high-low intensities of disrespect. Secondly, we investigate the impact of audio-based features such as pitch and intensity that can significantly elicit disrespect, and utilize the signals in classifying disrespect and non-disrespect samples by applying logistic regression model achieving 79.86% accuracy. Our findings shed light on the potential of utilizing audio-visual signals in adding important context towards understanding toxic discussions
Workshop Organizers
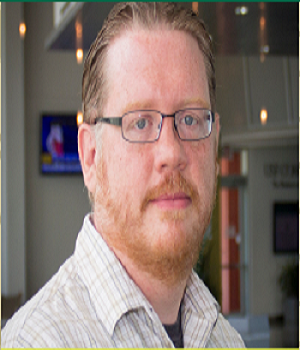
Shaun Canavan University of South Florida
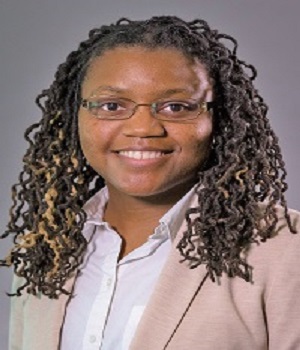
Tempestt Neal University of South Florida
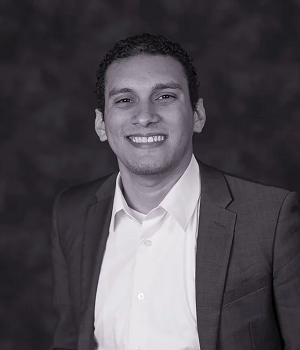
Marvin Andujar University of South Florida
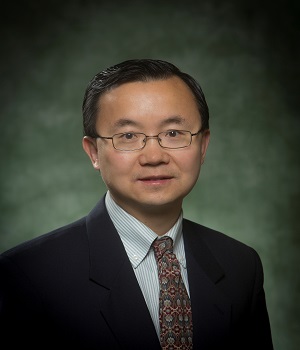