About The Workshop
Novel applications of affective computing have emerged in recent years in domains ranging from health care to the 5th generation mobile network. Many of these have found improved emotion classification performance when fusing multiple sources of data (e.g., audio, video, brain, face, thermal, physiological, environmental, positional, text, etc.). Multimodal affect recognition has the potential to revolutionize the way various industries and sectors utilize information gained from recognition of a person's emotional state, particularly considering the flexibility in the choice of modalities and measurement tools (e.g., surveillance versus mobile device cameras). Further, multimodal classification methods have been proven highly effective at minimizing misclassification error in practice and in dynamic conditions, and tend to be more stable over time compared to relying on a single modality, increasing their reliability in sensitive applications such as mental health monitoring and automobile driver state recognition. To continue the trend of lab to practice within the field and encourage new applications of affective computing, this workshop provides a unique forum for researchers to exchange ideas on future directions, including novel fusion methods and databases, innovations through interdisciplinary research, and emerging emotion sensing devices. Importantly, this workshop addresses the ethical use of novel applications of affective computing in real world scenarios, welcoming discussions on privacy, manipulation of users, and public fears and misconceptions regarding affective computing. This workshop seeks to explore the intersection between theory and ethical applications of affective computing, with a specific focus on multimodal data for affect recognition (e.g., expression and physiological signals) using pattern recognition, computer vision, and image processing.
Keynote Speakers

Dr. Hatice Gunes
Professor of Affective Intelligence & Robotics
Leader of Affective Intelligence and Robotics Lab
Department of Computer Science and Technology, University of Cambridge
Dr. Hatice Gunes is a Professor of Affective Intelligence and Robotics at University of Cambridge's Department of Computer Science and Technology. Prior to joining Cambridge in 2016, she was a Lecturer, then Senior Lecturer in the School of Electronic Engineering and Computer Science at Queen Mary University of London (QMUL), a postdoctoral researcher at Imperial College London, and an honorary associate of University of Technology, Sydney (UTS). Her research interests are in the areas of affective computing and social signal processing that lie at the crossroad of multiple disciplines including, computer vision, signal processing, machine learning, multimodal interaction and human-robot interaction. She has authored more than 125 papers in these areas (> 6,700 citations, H-index=36).

Dr. Stephanie Schuckers
Paynter-Krigman Endowed Professor in Engineering Science
Director of the Center for Identification Technology Research (CITeR)
Department of Electrical and Computer Engineering, Clarkson University
Dr. Stephanie Schuckers is the Paynter-Krigman Endowed Professor in Engineering Science in the Department of Electrical and Computer Engineering at Clarkson University and serves as the Director of the Center for Identification Technology Research (CITeR), a National Science Foundation Industry/University Cooperative Research Center. She received her doctoral degree in Electrical Engineering from The University of Michigan. Professor Schuckers research focuses on processing and interpreting signals which arise from the human body. Her work is funded from various sources, including National Science Foundation, Department of Homeland Security, and private industry, among others. She has started her own business, testified for US Congress, and has over 50 journal publications as well as over 100 other academic publications.
Workshop Schedule (EST)
9:00A-9:10A | Welcome and Opening Remarks |
9:15A-10:15A | Keynote 1 – Dr. Hatice Gunes |
10:15A-10:30A | Tyree Lewis, Rupal Agarwal and Marvin Andujar - An Ethical Discussion on BCI-Based Authentication |
10:30A-10:45A | Samuil Stoychev and Hatice Gunes - The Effect of Model Compression on Fairness in Facial Expression Recognition |
10:45A-10:55A | Break |
10:55A-11:10A | Yassine Ouzar, Lynda Lagha, Frédéric Bousefsaf and Choubeila Maaoui - Multimodal Stress State Detection from Facial Videos Using Physiological Signals and Facial Features |
11:10A-11:25A | Saandeep Aathreya and Shaun Canavan - Expression Recognition Using a Flow-Based Latent-Space Representation |
11:25A-12:25P | Keynote 2 – Dr. Stephanie Schuckers Biometric Recognition: Challenges and Possibilities In this ever increasing reliance on the digital society, biometric recognition is becoming a key pillar to establish and verify identity in order to ensure trust and prevent fraud. While the underlying functions of the technology perform sufficiently for purpose, challenges still remain, many that relate to the social-technology interface. This talk will highlight challenges including security, demographic variability, behavior, education, and responsible use. |
12:25P-12:30P | Closing Remarks |
Call for Papers
To investigate ethical, applied affect recognition, this workshop will leverage multimodal data that includes, but is not limited to, 2D, 3D, thermal, brain, physiological, and mobile sensor signals. This workshop aims to expose current use cases for affective computing and emerging applications of affective computing to spark future work. Along with this, this workshop has a specific focus on the ethical considerations of such work, including how to mitigate ethical concerns. Considering this, topics of the workshop will focus on questions including, but not limited to:
- What inter-correlations exist between facial affect (e.g. expression) and other modalities (e.g. EEG)?
- How can multimodal data be leveraged to create real-world applications of affect recognition such as prediction of stress, real-time ubiquitous emotion recognition, and impact of mood on ubiquitous subject identification?
- How can we facilitate the collection of multimodal data for applied affect recognition?
- What are the ethical implications of working on such questions?
- How can we mitigate the ethical concerns that such work produces?
- Can we positively address public fears and misconceptions regarding applied affective computing?
- Health applications with a focus on multimodal affect
- Multimodal affective computing for cybersecurity applications (e.g., biometrics and IoT security)
- Inter-correlations and fusion of ubiquitous multimodal data as it relates to applied emotion recognition (e.g. face and EEG data)
- Leveraging ubiquitous devices to create reliable multimodal applications for emotion recognition
- Applications of in-the-wild data vs. lab controlled
- Facilitation and collection of multimodal data (e.g. ubiquitous data) for applied emotion recognition
- Engineering applications of multimodal affect (e.g., robotics, social engineering, domain inspired hardware / sensing technologies, etc.)
- Privacy and security
- Institutionalized bias
- Trustworthy applications of affective computing
- Equal access to ethical applications of affective computing (e.g. medical applications inaccessible due to wealth inequality)
Workshop candidates are invited to submit papers up to 6 pages plus unlimited pages for references in the IEEE format. Submissions to AMAR 2022 should have no substantial overlap with any other paper submitted to ICPR 2022 or any paper already published. All persons who have made any substantial contribution to the work should be listed as authors (single-blind review). Papers presented at AMAR 2022 will appear in the IEEE Xplore digital library. Papers should follow the ICPR conference format (single-blind).
How to Submit:
Paper submissions will be handled using EasyChair.
Important dates:
Paper submission: June 6, 2022
Decision to Authors: June 20, 2022
Camera-ready papers due: September 1, 2022
Workshop: August 21, 2022
Accepted Papers
The Effect of Model Compression on Fairness in Facial Expression Recognition
Samuil Stoychev and Hatice GunesDeep neural networks are computationally expensive which has motivated the development of model compression techniques to reduce the resource consumption. Nevertheless, recent studies have suggested that model compression can have an adverse effect on algorithmic fairness, amplifying existing biases in machine learning models. With this work we aim to extend those studies to the context of facial expression recognition. To do that, we set up a neural network classifier to perform facial expression recognition and implement several model compression techniques on top of it. We then run experiments on two facial expression datasets, namely the Extended Cohn-Kanade Dataset (CK+DB) and the Real-World Affective Faces Database (RAF-DB), to examine the individual and combined effect that compression techniques have on the model size, accuracy and fairness. Our experimental results show that: (i) Compression and quantisation achieve significant reduction in model size with minimal impact on overall accuracy for both CK+ DB and RAFDB; (ii) in terms of model accuracy, the classifier trained and tested on RAF-DB is more robust to compression compared to the CK+ DB; and (iii) for RAF-DB, the different compression strategies do not increase the gap in predictive performance across the sensitive attributes of gender, race and age which is in contrast with the results on the CK+ DB where compression seems to amplify existing biases for gender.
Multimodal stress state detection from facial videos using physiological signals and facial features
Yassine Ouzar, Lynda Lagha, Frédéric Bousefsaf and Choubeila MaaouiStress is a complex phenomenon that affects the body and mind on multiple levels, encompassing both psychological and physiological aspects. Recent studies have used multiple modalities to comprehensively describe stress by exploiting the complementarity of multimodal signals. In this paper, we study the feasibility of fusing facial features with physiological cues on human stress state estimation. We adopt a multiple modalities fusion using a camera as a single input source and based on remote photoplethysmography method for non-contact physiological signals measurement. The frameworks rely on modern AI techniques and the experiments were conducted using the new UBFC-Phys dataset dedicated to multimodal psychophysiological studies of social stress. The experimental results revealed high performance when fusing facial features with remote pulse rate variability with an accuracy of 91.07%.
An Ethical Discussion on BCI-Based Authentication
Tyree Lewis, Rupal Agarwal and Marvin AndujarIn recent times, Brain Computer Interface (BCI) applications have progressed as biometric authentication systems. Existing systems each follow the fundamental concepts of ensuring a user can be successfully authenticated into it. This comes with the complexity of developing systems that prevent a user’s data from being at risk. Furthermore, with each new integration to make these systems more secure, they may lead to ethical concerns that should be discussed to better understand if they will be beneficial to individuals in their daily life. In this paper, we present an ethical discussion of a BCI-based authentication system, that has the potential to combat the risks that occur in other forms of authentication systems. We additionally provide insight as to the ethical issues that can occur, so that it can be effective for everyone to utilize.
Expression Recognition using a Flow-based Latent-space Representation
Saandeep Aathreya and Shaun CanavanFacial expression Recognition is a growing and important field that has applications in fields such as medicine, security, education, and entertainment. While there have been encouraging approaches that have shown accurate results on a wide variety of datasets, in many cases it is still a difficult problem to explain the results. To enable deployment of expression recognition applications in-the-wild, being able to explain why an particular expression is classified is an important task. Considering this, we propose to model flow-based latent representations of facial expressions, which allows us to further analyze the features and grants us more granular control over which features are produced for recognition. Our work is focused on posed facial expressions with a tractable density of the latent space. We investigate the behaviour of these tractable latent space features in the case of subject dependent and independent expression recognition. We employ a flow-based generative approach with minimal supervision introduced during training and observe that traditional metrics give encouraging results. When subject independent expressions are evaluated, a shift towards a stochastic nature, in the probability space, is observed. We evaluate our flow-based representation on the BU-EEG dataset showing our approach provides good separation of classes, resulting in more explainable results.
Workshop Organizers

Shaun Canavan University of South Florida
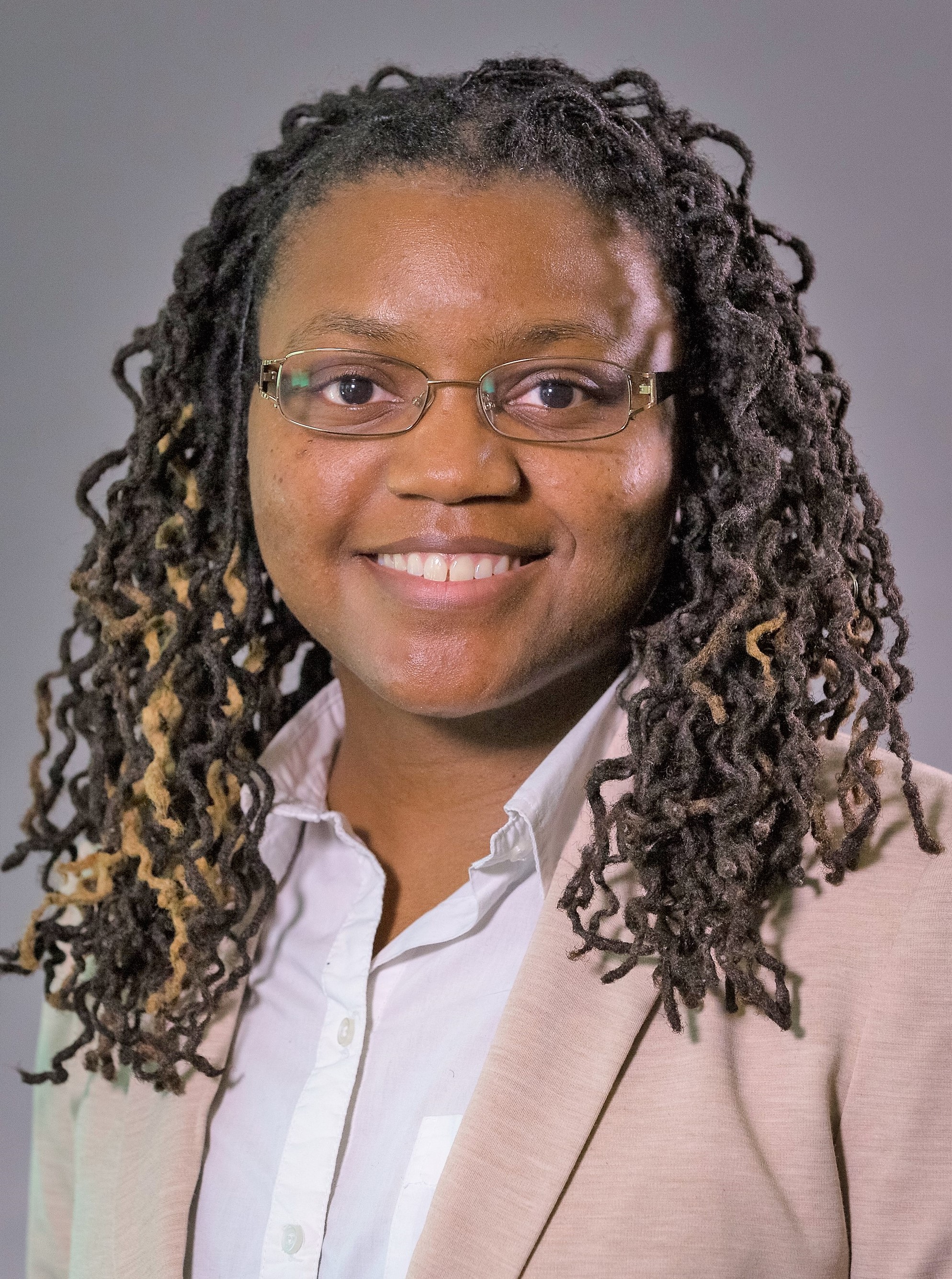
Tempestt Neal University of South Florida

Marvin Andujar University of South Florida

Saurabh Hinduja University of Pittsburgh

Lijun Yin Binghamton University
Program Committee
- Kalaivani Sundararajan, Koh Young Research Canada
- David Crandall, Indiana University Bloomington
- Sayde King, University of South Florida
- Khadija Zanna, Rice University
- Nima Karimian, San Jose State University
- Huiyuan Yang, Rice University
- Venkata Sri Chakra Kumar, Cornell University