About The Workshop
In recent years, the landscape of affective computing has witnessed remarkable advancements, finding applications in diverse domains such as healthcare and 5th generation mobile networks. Notably, the integration of multiple data sources, including audio, video, brain activity, facial expressions, thermal readings, physiological signals, environmental cues, positional data, and text, has significantly enhanced emotion classification performance. The flexibility in choosing modalities and measurement tools, such as surveillance or mobile device cameras, opens up avenues for revolutionary changes across various industries. Multimodal affect recognition, with its proven effectiveness in minimizing misclassification errors, emerges as a key player in applications ranging from mental health monitoring to automobile driver state recognition. This workshop serves as a pivotal platform for researchers to collaborate and exchange ideas on the future directions of affective computing. Topics of discussion include novel fusion methods, database innovations, interdisciplinary research breakthroughs, and emerging emotion-sensing devices. Recognizing the ethical implications of these advancements, the workshop delves into crucial issues like privacy, user manipulation, and public concerns surrounding affective computing. The anticipated growth of the affective computing market, projected to reach $140 billion by 2025, underscores the potential for groundbreaking applications. This workshop aims to explore the intersection between theoretical frameworks and ethical applications of affective computing, with a specific emphasis on multimodal data for affect recognition, including facial expressions and physiological signals. Building on the success of previous workshops, such as the Ubiquitous Emotion Recognition with Multimodal Mobile Interfaces at UbiComp 2018 and the first three editions of this workshop (AMAR) at FG 2020, ACII 2021, and ICPR 2022, the 4th AMAR workshop continues its focus on ethical applications of affect recognition using pattern recognition, computer vision, and image processing. Join us in shaping the future of affective computing, where innovation meets responsibility, and theory converges with ethical practice.
Keynote Speaker

Dr. Jeffrey Cohn
Professor of Psychology, Psychiatry, and Intelligent Systems, University of Pittsburgh
Adjunct Professor of Computer Science, Robotics Institute, Carnegie Mellon University
Chief Scientist and Co-Founder, Deliberate.ai
Dr. Jeffrey Cohn leads interdisciplinary and inter-institutional efforts to develop advanced methods of automatic analysis and synthesis of facial expression and prosody and applies those tools to research in human emotion, social development, nonverbal communication, psychopathology, and biomedicine. His research has been supported by grants from the U.S. National Institutes of Health, National Science Foundation, Autism Foundation, Office of Naval Research, and Defense Advanced Research Projects Agency among other sponsors. He is a scientific advisor for RealEyes and for Embodied, past Chair of the Steering Committee of the IEEE International Conference on Automatic Face and Gesture Recognition (FG) from 2011-2021, a Fellow of the Association for the Advancement of Affective Computing. He has co-edited IEEE Transactions in Affective Computing (TAC) and special issues on affective computing for IEEE Transactions in Pattern Analysis and Machine Intelligence, Journal of Image and Vision Computing, Pattern Recognition Letters, Computer Vision and Image Understanding, and ACM Transactions on Interactive Intelligent Systems.

Sayde King
Ph.D. Candidate, Department of Computer Science and Engineering, College of Engineering, University of South Florida
Sayde King is a Ph.D. Candidate in the Department of Computer Science and Engineering at the University of South Florida. She is also a member of the Cyber Identity and Behavior Research Laboratory, which studies biometric systems, smart sensing for human behavior analyses, and applied natural language processing. Her research explores automated deception detection for non-engineering applications. Ms. King received her M.S. and B.S. in Computer Science from the University of South Florida in 2022 and 2019, respectively. She has actively contributed to the academic community by serving as a program committee member for workshops at renowned conferences such as the IEEE International Conference on Automatic Face and Gesture Recognition and the IEEE/IAPR International Conference on Pattern Recognition. She is a Florida Education Fund McKnight Dissertation Fellow, Alfred P. Sloan Foundation Minority Ph.D. Scholar, and a National GEM Consortium Employer Fellow, sponsored by the MIT Lincoln Laboratory.
Workshop Schedule (Tentative)
Time | Activity |
---|---|
9:15AM - 9:30AM | Welcome and Opening Remarks from Organizers |
9:30AM - 10:30AM | Keynote |
10:30AM - 10:45AM | Workshop Paper Presentation |
10:45AM - 11:00AM | Coffee Break |
11:00AM - 11:15PM | Workshop Paper Presentation |
11:15AM - 12:15PM | Keynote |
12:15PM - 12:30PM | Closing Remarks |
Call for Papers
This workshop will explore ethical considerations in applied affect recognition, fostering conversations on topics utilizing multimodal data, including but not limited to 2D, 3D, thermal, brain, physiological, and mobile sensor signals. The goal is to highlight current use cases for affective computing and identify emerging applications, stimulating future research. The workshop is designed for researchers across various fields, including Brain-Computer Interfaces (BCI), affective computing, biometrics, computer vision, human-computer interaction, behavioral sciences, social sciences, and policymakers.
The workshop will focus on questions such as:
- What inter-correlations exist between facial affect (e.g., expression) and other modalities (e.g., EEG, fNIRS)?
- How can multimodal data be used in real-world applications of affect recognition, such as predicting stress, real-time ubiquitous emotion recognition, and understanding the impact of mood on ubiquitous subject identification?
- How can we facilitate the collection of multimodal data for applied affect recognition?
- What are the ethical implications of working on such questions?
- How can we mitigate the ethical concerns that such work produces?
- Can we positively address public fears and misconceptions regarding applied affective computing?
- Health applications with a focus on multimodal affect
- Multimodal affective computing for cybersecurity applications (e.g., biometrics and IoT security)
- Inter-correlations and fusion of ubiquitous multimodal data as it relates to applied emotion recognition (e.g. face and EEG data)
- Leveraging ubiquitous devices to create reliable multimodal applications for emotion recognition
- Applications of in-the-wild data vs. lab controlled
- Facilitation and collection of multimodal data (e.g. ubiquitous data) for applied emotion recognition
- Engineering applications of multimodal affect (e.g., robotics, social engineering, domain inspired hardware / sensing technologies, etc.)
- Privacy and security
- Institutionalized bias
- Trustworthy applications of affective computing
- Equal access to ethical applications of affective computing (e.g. medical applications inaccessible due to wealth inequality)
Authors are invited to submit long and short papers in the IEEE format. Submissions to AMAR2024 should have no substantial overlap with any other paper submitted to FG 2024 or any paper already published. Papers presented at AMAR2024 will appear in the IEEE Xplore digital library. Papers must follow the FG paper format (double-blind).
How to Submit:
Paper should be submitted to EasyChair for review.
Important dates:
Paper submission: March 28, 2024
Decision to Authors: April 12, 2024
Camera-ready papers due: April 22, 2024
Workshop: TBA
Accepted Papers
- "Mitigating Class Imbalance for Facial Expression Recognition using SMOTE on Deep Features." Tara Nourivandi, Saurabh Hinduja, Shivam Srivastava, Jeffrey Cohn and Shaun Canavan.
Applications of affective computing are used in high-stake decision-making systems. Many of these systems that have applications in medicine, education, entertainment, and security user facial expression recognition (FER). Because of the significance of these applications, fair, unbiased, generalizable, and valid systems are essential. A source of error in FER can be class imbalance, which leads to bias against labels that occur less frequently. Considering this, we propose a solution to mitigate class imbalance for FER by synthesizing new deep features utilizing the Synthetic Minority Oversampling Technique (SMOTE) on deep features extracted from a neural network. We refer this to as a SMOTE layer in a neural network. More specifically, we up-sample the deep features of each minority class so that classes are balanced during training. To validate the efficacy of the proposed approach, we perform experiments on the publicly available datasets BP4D and AffectNet. We show encouraging results on BP4D, for pain recognition from facial expressions, and on AffectNet for 8-class classification of facial expressions. - "Toward Emotion Recognition and Person Identification Using Lip Movement from Wireless Signals: A Preliminary Study." Sayde King, Mohamed Ebraheem, Phuong Dang and Tempestt Neal.
We present a first of its kind pilot study investigating distinct features presented in lip movement captured through WiFi channel state information (CSI) as four research volunteers read several emotion-charged text samples. We pursue the tasks of emotion and identity recognition with these data using statistical and frequency-domain features. While the extracted frequency-domain features, i.e., zero-crossing rate and fundamental frequency, are commonly associated with audio and speech recognition related applications, we found statistical features, such as mean, median, skew, and kurtosis, most suitable for capturing salient information in CSI data. Specifically, classifying the emotional states of speakers (i.e., anger, joy, fear, love, surprise, and sadness) and the identity of speakers themselves, we achieved 96.4% and 57.9% accuracy for the identity and emotion recognition tasks, respectively.
Workshop Organizers

Shaun Canavan University of South Florida
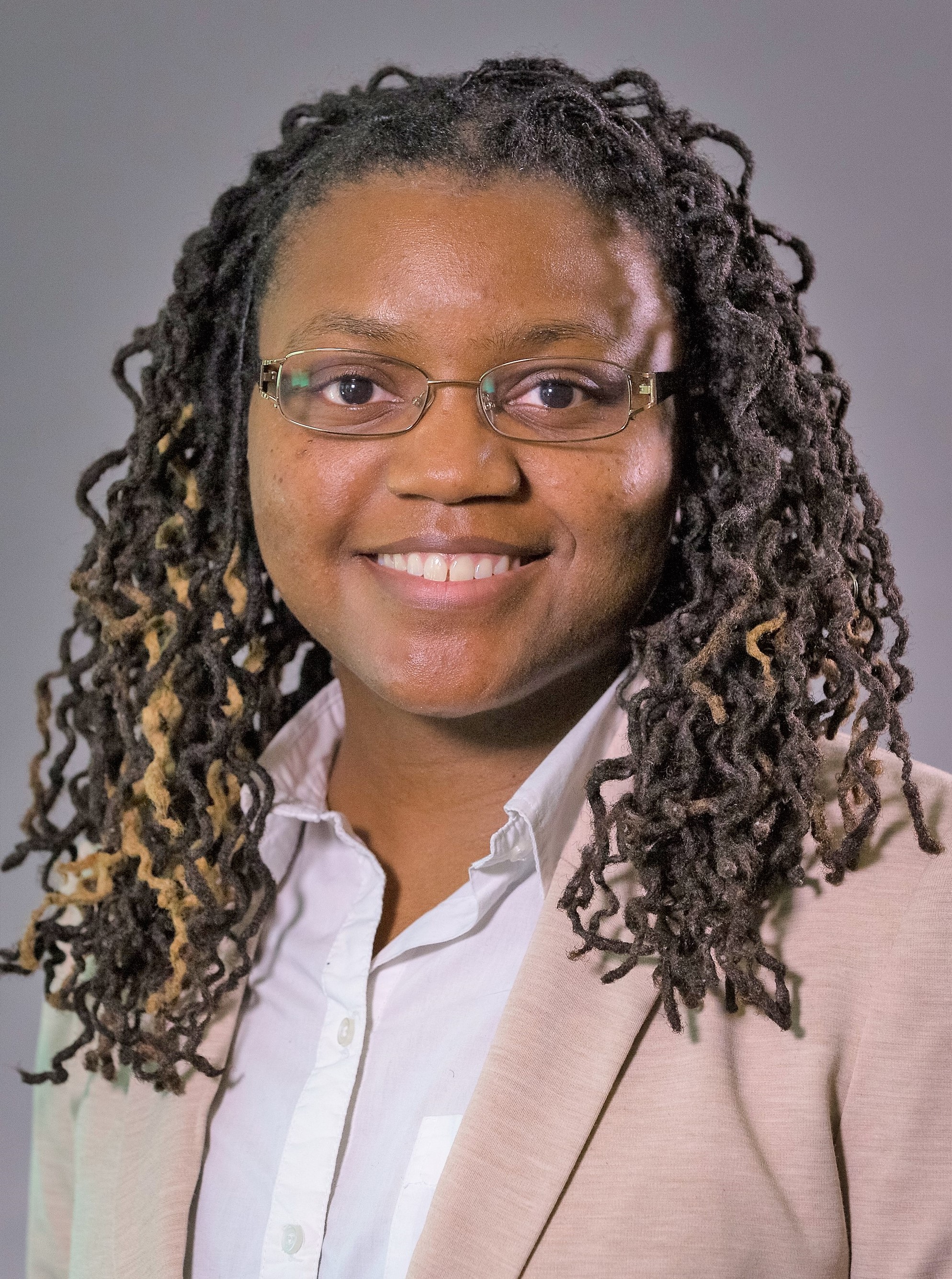
Tempestt Neal University of South Florida

Marvin Andujar University of South Florida

Saurabh Hinduja University of Pittsburgh
